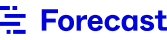
Capacity modeling is a term that's getting more attention in business and technology circles—and for good reason. As organizations strive to operate efficiently and avoid unnecessary costs, capacity modeling provides a data-driven way to ensure resources align with demand. In this FAQ, we’ll explore the basics, uses, benefits, and best practices of capacity modeling. Here’s everything you need to know about capacity modeling to see how it might improve decision-making in your organization.
Capacity modeling is a process that helps organizations forecast their resource needs to meet demand without over- or under-utilizing their assets. It involves analyzing data on current and projected workload, resources, and constraints to predict how much capacity (such as labor, equipment, or computing power) will be needed in the future. For example, companies can use capacity modeling to predict staffing needs, plan for production or service delivery, and allocate IT resources to handle fluctuating workloads. Capacity modeling ensures that organizations neither overcommit (leading to wasted resources) nor underprepare (leading to unmet demand), creating a balanced approach to resource management.
Capacity modeling is crucial because it helps organizations optimize resource allocation, reduce costs, enhance customer satisfaction, and improve strategic planning. By accurately predicting resource requirements, businesses can allocate resources efficiently, preventing unnecessary expenditures on excess capacity or the expensive consequences of unmet demand. When resources are in place to meet demand, businesses can deliver products or services more reliably. Capacity modeling informs both short- and long-term planning by revealing trends in resource demand. In industries like manufacturing, IT, and healthcare, capacity modeling can make a significant difference in operational effectiveness and cost savings.
Capacity modeling can take various forms depending on the organization's needs. Predictive modeling uses historical data to forecast future resource requirements. Simulation modeling simulates different scenarios to see how changes in demand or resources would affect capacity. Scenario planning examines various “what if” scenarios to plan for uncertain future demands. Constraint-based modeling considers constraints such as budget, space, or time when forecasting capacity. Each type of modeling offers unique insights, and organizations often use a combination to get a complete view of their capacity needs.
Capacity modeling typically involves a few key steps. First, data collection is essential to gather information on current resources, workloads, demand trends, and other relevant factors. Then, organizations define assumptions about future demand, growth rates, and constraints. Using predictive or simulation software, they create a model that reflects expected capacity needs. After creating the model, they analyze the output to determine resource requirements and identify any potential gaps. Capacity models are rarely static, so regular updates are needed as new data and trends emerge. Software tools often help streamline this process by automating parts of data collection, analysis, and reporting.
Capacity modeling is widely used across industries, especially where demand fluctuates and efficient resource use is critical. In manufacturing, capacity modeling helps manage production lines, inventory, and staff to meet fluctuating demand. IT and cloud services use capacity modeling to allocate servers, storage, and bandwidth in response to changing data processing needs. In healthcare, it ensures adequate staffing and resources, especially in emergency services. Retail uses capacity modeling for managing inventory and staffing during peak seasons like holidays.
Capacity modeling can be challenging for several reasons. Poor or incomplete data can lead to inaccurate models. Shifts in market demand or customer behavior make it difficult to predict needs accurately. Limited budgets or physical resources can limit the scope of capacity solutions. Multiple factors, like weather or economic changes, can impact capacity needs in unpredictable ways. Organizations can mitigate these challenges with better data collection, periodic model updates, and cross-departmental collaboration.
Capacity modeling and capacity planning are related but distinct concepts. Capacity modeling is the process of creating a model to predict capacity needs based on various factors, while capacity planning involves using the model’s predictions to make actual decisions and implement changes in resource allocation. In essence, capacity modeling is part of the larger capacity planning process, which may include procurement, budgeting, and personnel management.
There are several software tools designed to aid capacity modeling, including Microsoft Excel, which is often used for simple capacity models with fewer variables. Simulation software, like AnyLogic or Simio, helps simulate complex scenarios. Enterprise Resource Planning (ERP) tools like SAP or Oracle include capacity modeling features. Cloud-based tools like AWS CloudWatch or Microsoft Azure Monitor are common for IT and cloud resource management. The choice of tool depends on the complexity of the model, the industry, and the specific needs of the organization.
Artificial Intelligence (AI) is becoming increasingly valuable in capacity modeling. AI can analyze large amounts of data and identify patterns that may be too complex for traditional analysis. It enables dynamic modeling, allowing for real-time adjustments to capacity models based on new data. AI also improves accuracy as machine learning algorithms continuously refine the model to improve prediction accuracy over time. AI makes capacity modeling more efficient, adaptive, and insightful, especially for large-scale operations with complex data inputs.
To ensure success, organizations should follow these best practices: use quality data to build reliable models, regularly update models as demand patterns change, involve stakeholders from various departments to improve the comprehensiveness of capacity models, start with straightforward models and add complexity as experience grows, and leverage software to automate data collection and model adjustments, saving time and improving accuracy.
Yes, small businesses can also benefit from capacity modeling. By predicting when demand may exceed capacity, even small businesses can make smarter hiring, inventory, and purchasing decisions. Tools like Excel, Google Sheets, or cloud-based platforms make capacity modeling accessible and cost-effective for small to medium enterprises (SMEs) as well.
Capacity models should be updated based on the industry and volatility of demand. For fast-changing environments like tech or retail, monthly or quarterly updates might be necessary. In more stable sectors, an annual review may be sufficient. Consistent updates ensure that models accurately reflect current conditions, helping businesses stay agile.
Capacity modeling is an essential practice for businesses looking to stay competitive and resource-efficient. By understanding demand and aligning resources accordingly, organizations can cut costs, increase productivity, and deliver better services. With the right tools and strategies, capacity modeling can be a valuable asset for organizations of all sizes.
The ultimate upgrade to delivering projects on time and on budget.
Request a Demo